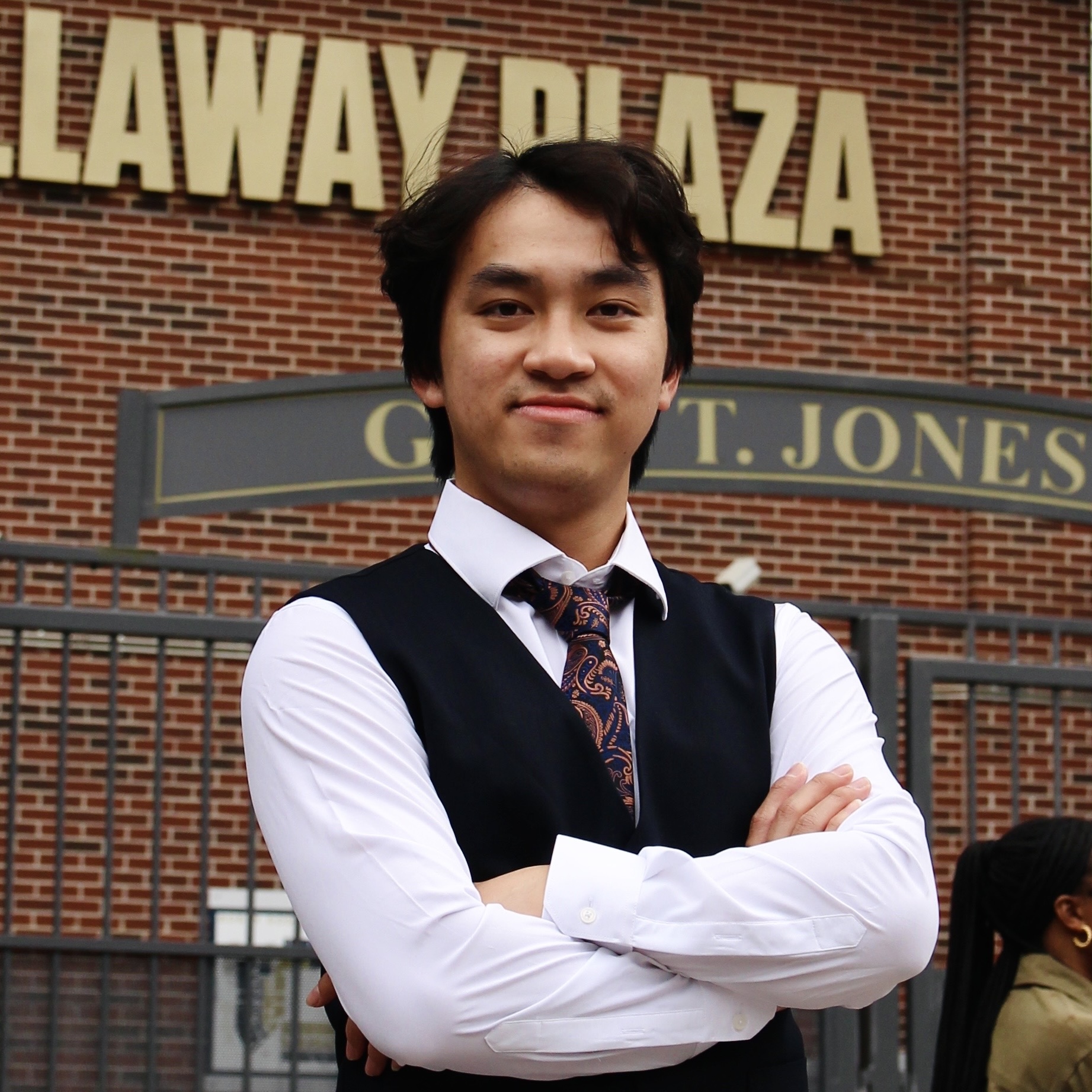
Quan Nguyen
PhD Student,
Children's Hospital of Philadelphia
Quan Minh Nguyen is a Bioengineering PhD student at the University of Pennsylvania, working under the mentorship of Dr. Kai Wang at the Children's Hospital of Philadelphia. His research focuses on developing advanced data mining approaches to analyze clinical phenotypic information in Electronic Health Records (EHR), with the goal of correlating genotype and phenotype to unravel the phenotypic heterogeneity of inherited diseases. With a concentration in artificial intelligence (AI) and large language models (LLMs), Quan specializes in designing computational solutions for medical applications.
As part of his work, he has been developing and improving AI tools such as PhenoGPT and Phen2Test. These tools employ machine learning and natural language processing to extract clinical phenotypes from unstructured EHR data, enabling the prediction of genetic syndromes and candidate genes. By integrating LLMs and advanced AI techniques, Quan's research promises to advance precision medicine and facilitate more accurate, early diagnosis of rare and complex genetic disorders.
Quan's primary research interest lies in developing a multimodality model that integrates diverse data sources, including clinical texts, demographic information, medical imaging, and genomic data. By unifying these heterogeneous datasets, Quan aims to create a comprehensive framework capable of capturing complex interactions between various biological and clinical factors. This innovative approach has the potential to enhance disease diagnosis, prognosis, and personalized treatment strategies. He leverages LLM techniques, such as knowledge graph integration, retrieval-augmented generation (RAG), chain-of-thought (CoT) prompting, and fine-tuning, to enhance the interpretability and performance of LLMs in biomedical applications. By incorporating domain-specific knowledge and multimodal data into these models, he ensures more accurate, context-aware insights for clinical decision-making. His work aims to push the boundaries of LLM capabilities, particularly in synthesizing complex healthcare information for precision medicine.
For collaborations or inquiries, connect with Quan on LinkedIn at www.linkedin.com/in/quan-nguyen-103 or via email at qmn103@seas.upenn.edu.
As part of his work, he has been developing and improving AI tools such as PhenoGPT and Phen2Test. These tools employ machine learning and natural language processing to extract clinical phenotypes from unstructured EHR data, enabling the prediction of genetic syndromes and candidate genes. By integrating LLMs and advanced AI techniques, Quan's research promises to advance precision medicine and facilitate more accurate, early diagnosis of rare and complex genetic disorders.
Quan's primary research interest lies in developing a multimodality model that integrates diverse data sources, including clinical texts, demographic information, medical imaging, and genomic data. By unifying these heterogeneous datasets, Quan aims to create a comprehensive framework capable of capturing complex interactions between various biological and clinical factors. This innovative approach has the potential to enhance disease diagnosis, prognosis, and personalized treatment strategies. He leverages LLM techniques, such as knowledge graph integration, retrieval-augmented generation (RAG), chain-of-thought (CoT) prompting, and fine-tuning, to enhance the interpretability and performance of LLMs in biomedical applications. By incorporating domain-specific knowledge and multimodal data into these models, he ensures more accurate, context-aware insights for clinical decision-making. His work aims to push the boundaries of LLM capabilities, particularly in synthesizing complex healthcare information for precision medicine.
For collaborations or inquiries, connect with Quan on LinkedIn at www.linkedin.com/in/quan-nguyen-103 or via email at qmn103@seas.upenn.edu.
Sessions
-
21-Mar-2025Los Angeles Convention CentrePlatform Session 7: Genetic Data, Health Services, and ELSI